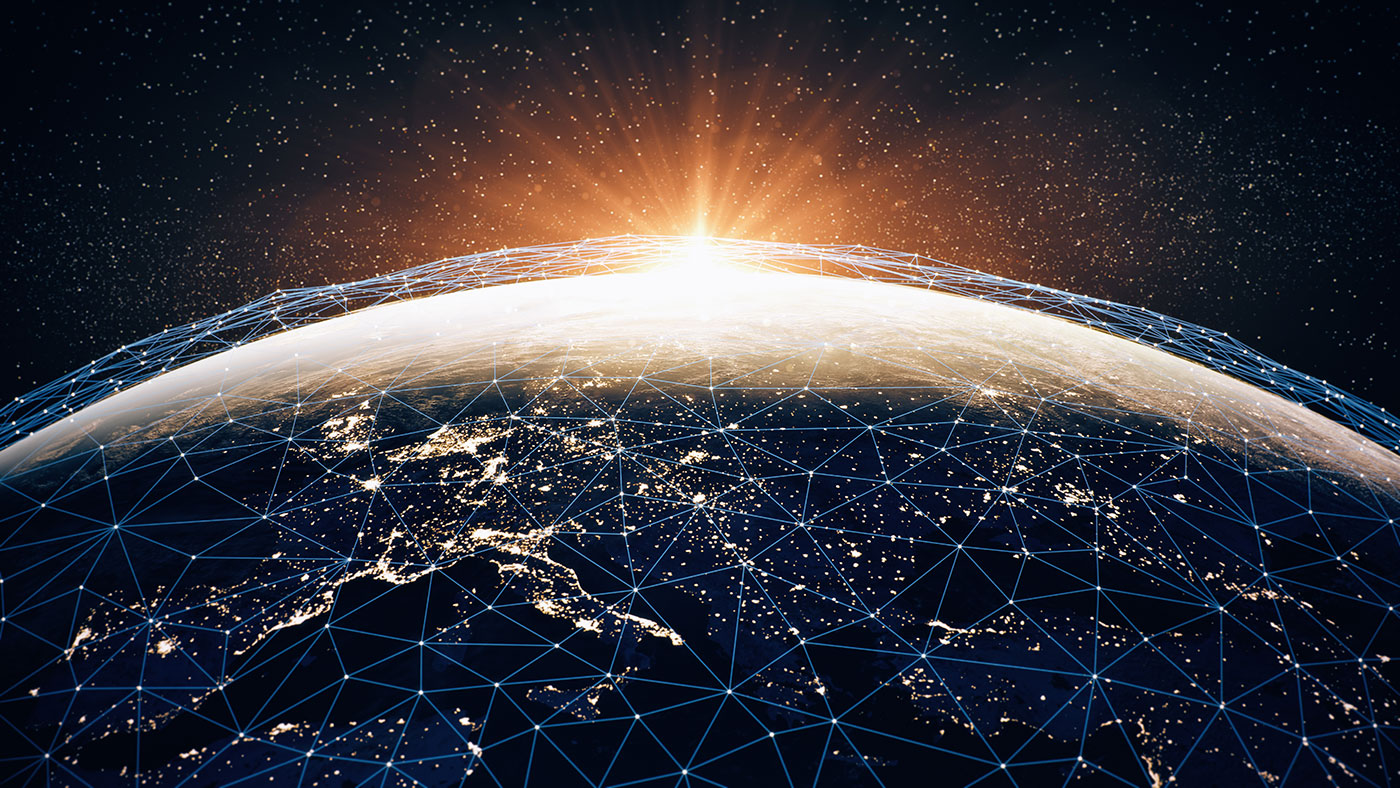
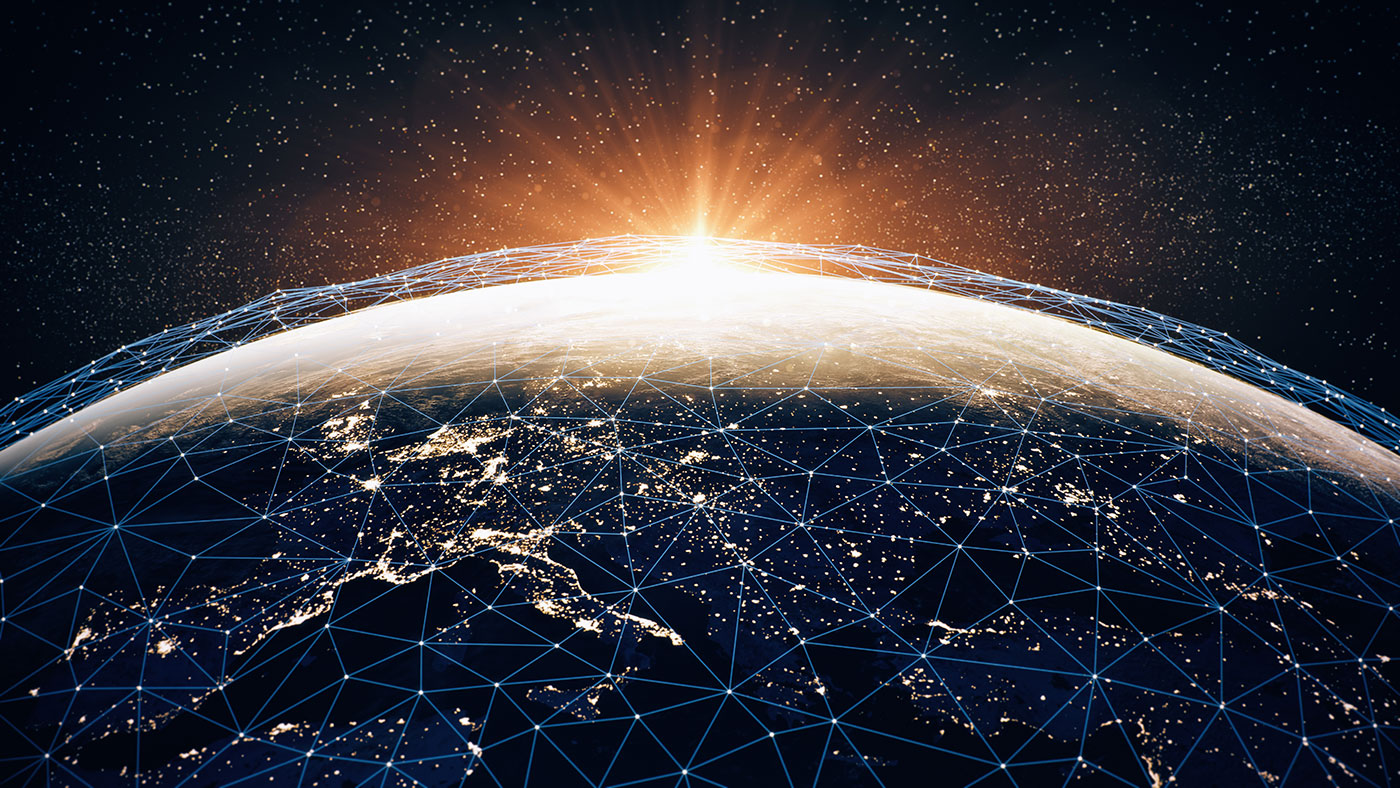
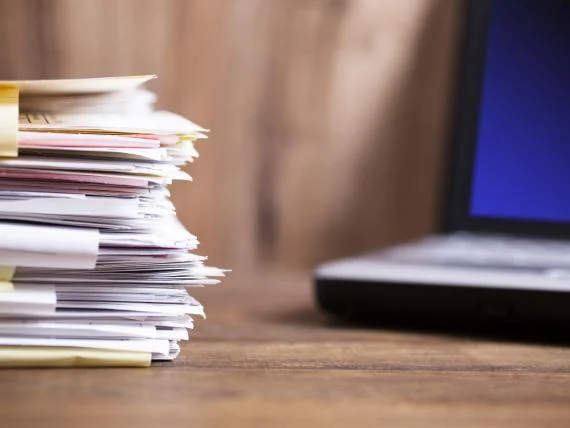
Learning from incidents and accidents is essential to preventing them from reoccurring. Often, the best lessons hide within SIFs (Serious Injuries or Fatalities) which have to be reported under the RIDDOR (Reporting of Injuries, Diseases and Dangerous Occurrences Regulations 2013) legislation. However, identifying them is a laborious and time-consuming task requiring safety professionals to wade through a sea of lengthy safety reports.
Being able to instantly recognise when an incident needs to be reported would be more efficient. It would allow safety professionals to analyse unprecedented quantities of safety data, vastly enhancing their ability to analyse the causes of incidents and accidents, and advise industry on what to do to prevent the same thing from happening again.
This is the potential of the ‘Severity Scanner’ we’re developing as a pilot project with Lloyd’s Register and industry partner Nando’s and Mitchell’s and Butler’s (M&B), the name behind many of the UK’s best-known restaurant chains including Harvester and Toby’s Carvery. M&B have over 1,700 venues across the UK, generating vast quantities of incident data.
Ran Merkazy, VP, Product and Services Innovation at Lloyds Register, said: “Working on the pilot with Mitchell and Butler highlighted the burden placed on companies’ resources of reviewing every incident that happens to see if it needs to be reported. The estimate is that reviewing all the data for reportable SIFs takes one full-time employee’s time. Additionally, human error is always an issue, leaving companies exposed to the risk of fines and investigations, due to unreported RIDDORs.”
The Severity Scanner harnesses the power of machine learning and natural language processing (NLP) to ‘listen at scale’, by mining through vast quantities of safety reports and flagging which incidents are reportable under RIDDOR. NLP is a sub-field of artificial intelligence (AI) that uses machines’ increasing ability to understand written language almost as a human being would. This enables them to read through free text rather than searching for keywords.
“AI doesn’t replace human safety expertise – it only does what an expert can do, but at scale,” says Ran. “The way we use it is through a combination of predetermined training methods (supervised learning) and machine-learning techniques (unsupervised).”
“A good example would be to compare it to keyword search. There are different words that mean the same thing, which a keyword search cannot scan for. Then there are the unknowns, where you are not even sure what you are looking for – where are the severity hot spots? Both of these issues are answered by NLP’s capabilities to see beyond phrase and into meaning, and to identify hidden clusters.”
Another advantage of the AI ‘Scanner’ is the ability to look into the past, to identify further clusters and trends. It’s currently being applied across the vast library of safety reports held by the HSE, holding information on almost every conceivable type of incident and accident. The system will learn as it develops, able to draw on this knowledge in quickly scanning new safety reports to tell users whether they need to report an incident under RIDDOR.
The ability to scan, process and assess vast quantities of text in this way will empower safety professionals to make much better informed decisions feeding into root cause analysis.
“We’ve taken vast amounts of severe incident reports,” explains Ran, “and used them to train an algorithm to automatically identify passages that describe a similar incident. We ended up with a set of 23 different severe incident classifiers, that can now be accurately used to map out a data set and alert users to severe incidents they haven’t noticed.”
The Severity Scanner will improve efficiency by helping organisations avoid the human cost of complying with legislation. It will also help them learn from each other, by providing an anonymised resource where organisations can learn from shared RIDDOR reports.
The Severity Scanner will be embedded within a larger system, Lloyd’s Register’s Safety Scanner, which automates analysis of safety data and brings ‘listening at scale’ to organisations that are swamped with human input in the form of natural language. The pilot project has been designed to show whether the algorithm inside the tool works effectively in analysing the severity of incidents.
“This is about creating a pool of data on a scale never seen before,” says Ran, “and using the latest in AI to mine it for valuable safety insights. We are combining more than 40 years of data from the HSE with other sources around the world, and the pilot with Mitchell and Butler is teaching us a lot about how businesses can use these insights to support their operations.”
The next stage will be to expand the algorithm’s training using data from outside the hospitality sector. Discovering Safety is now looking for early adopters – companies or organisations from other industries – to help us develop this further. To register your interest, email discoveringsafety@hse.gov.uk
Related Content