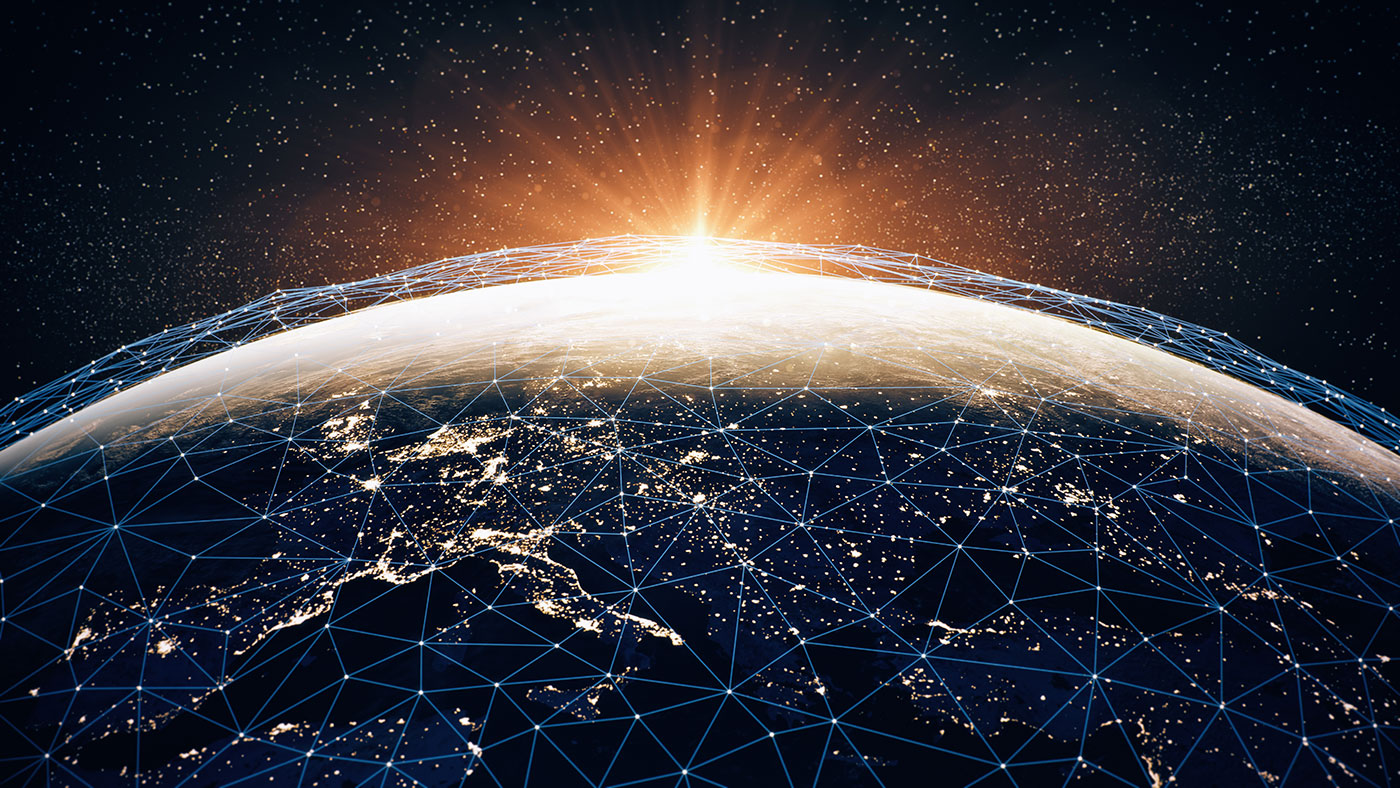
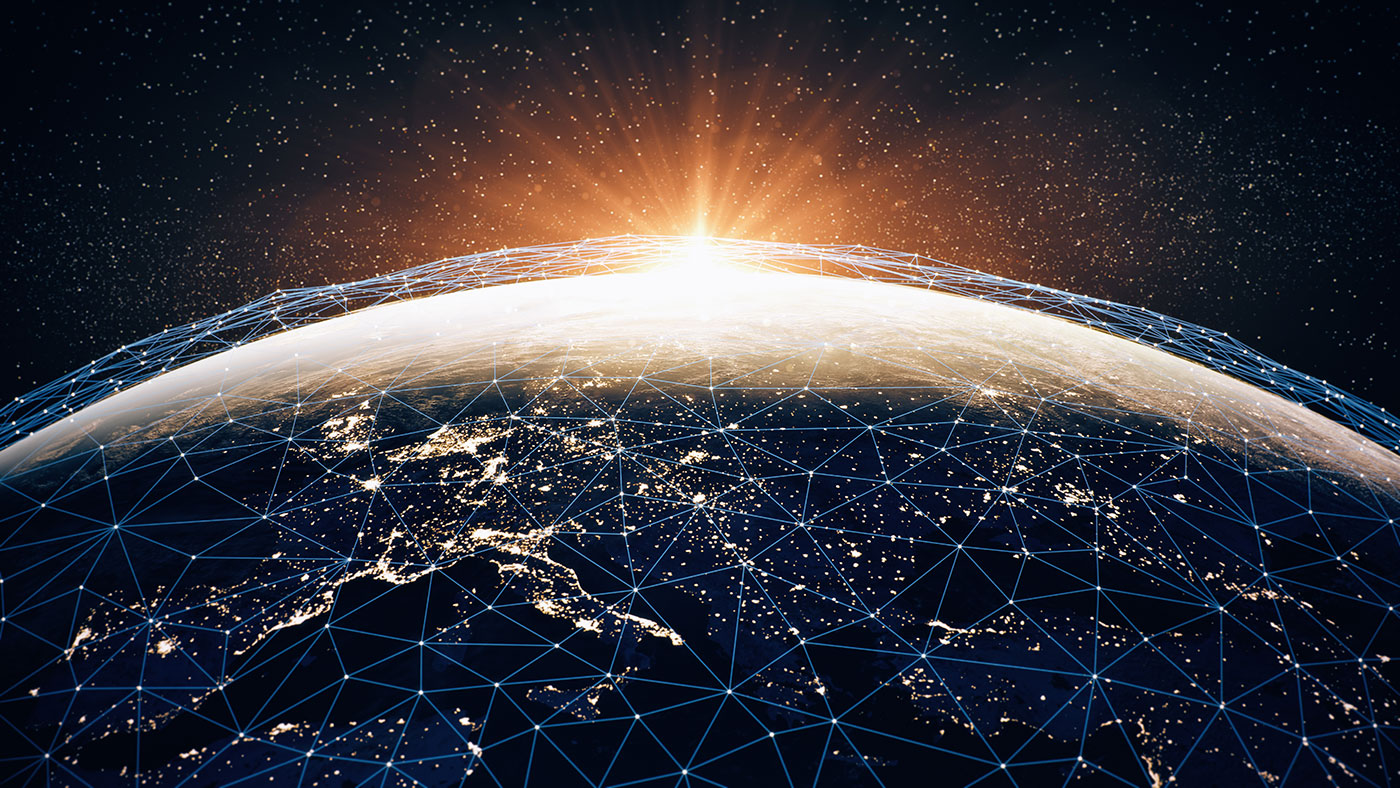
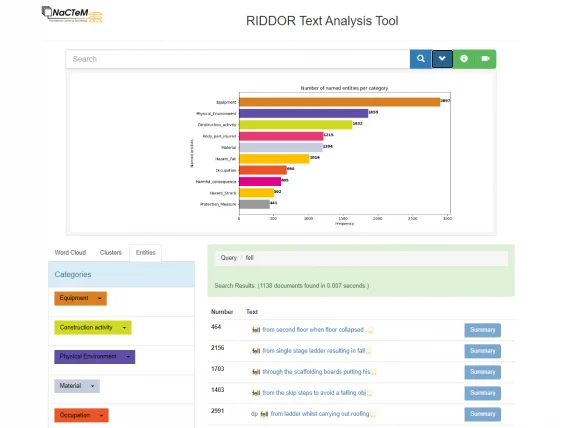
A new text search system has the potential to boost safety planning on construction sites. It has been developed by the Health and Safety Executive (HSE) in collaboration with the National Centre for Text Mining, University of Manchester (NaCTeM).
The RIDDOR Text Analysis Tool is a critical element of the Discovering Safety Programme (a collaboration between the HSE and Lloyd’s Register Foundation, who are also funders). It’s been developed following discussions with the construction industry – still, in spite of decades of advances in health and safety, the source of many workplace accidents. Forty UK construction workers lost their lives because of work-related harm in 2019-2020. That’s on top of 81,000 people living with ill-health suffered at work.
As its name suggests, the RIDDOR Text Analysis Tool is based on the accident reports submitted to HSE under the Reporting of Injuries, Diseases and Dangerous Occurrences Regulations (RIDDOR) 2013. It uses natural language processing and machine learning to perform semantic searching on accident and incident textual data. It’s available to view on the NaCTeM website (where there is also a helpful video).
Information from RIDDOR reports and other safety documents is vital for industry in the quest to prevent people from being harmed at work – but until now, trawling through the vast volume of information generated by accidents has been a slow, laborious task. Until now it has only been possible to search through RIDDOR reports using ‘codified data’ – terms such as type of injury or the age of the person who’s been injured. This then brings up a long list of documents that have to be read through to get to the relevant facts.
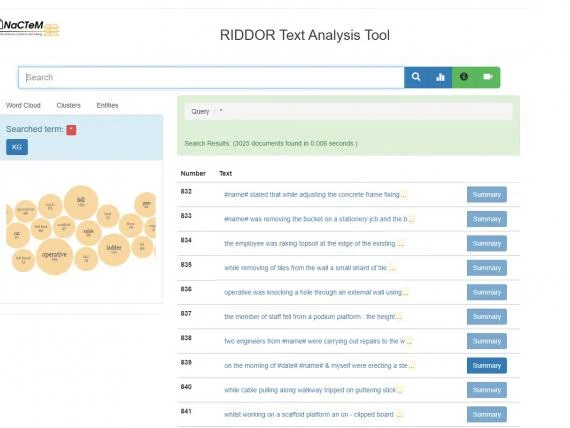
The tool is a big step forward. By training computers how to read text almost like a human, NaCTeM’s experts have created a system that can do in-depth analysis instantly. It ‘mines’ through the free text of the HSE documents to explore the contents and present the relevant information in an instantly usable form.
This will enable health and safety managers, contractors and HSE inspectors to extract pertinent safety-critical concepts and associations without the labour of trawling through thousands of pages of text. It brings together HSE data and the power of artificial intelligence – in particular natural language processing and deep learning – to make devising risk assessments more accurate, effective, intuitive and much easier.
“This will help users carrying out risk assessments by quickly providing insights – for example, before using a piece of equipment for the first time,” explained Tim Yates, HSE data scientist. Tim worked closely with NaCTeM on developing the tool.
By proving that applying Natural Language Processing (NLP) tools to safety data really works – performance of the backend NLP tools has accuracy of up to 90% compared to traditional keyword approaches – the tool opens the door to new, more efficient searching of valuable health and safety information for all kinds of industries.
Professor Sophia Ananiadou, Director of NaCTeM, Department of Computer Science, led the team which developed the tool. She explains:
“It makes sense of large volumes of textual data in an efficient and intuitive manner; it gets right to the heart of what the searcher is looking for. In an industry where risk management is crucial, this is a solution to the problem of overlooking risks due to the overwhelming amount of text.
“The tool allows users to drill down in an interactive manner and easily find the information they need. If that’s guidance on what to do to prevent falls, it will present a set of short summaries, with key words (such as ‘fall’, ‘ankle’ or ‘ladder’) highlighted, giving immediate access to the relevant information. This could include what happened, what needs to happen to prevent any further occurrences and any protective measures taken.”
If you enter ‘ladder’ and ankle into the search engine, the tool instantly brings up clusters of words gathered from reports containing them – and others such as ‘slab’ ‘kerb’ and ‘slipped’ that guide you to think more widely around the subject. Alongside this is a list of 587 documents (found in 0.007 seconds) – but presented with relevant extracts such as ‘ladder gave way, slipped along the plane of elevation’ rather than just allowing you to download the full document.
Tim explains the potential of the RIDDOR Text Analysis Tool for the construction contractor, looking for information on a particular type of accident or incident with a view to preventing it from happening in the future:
“The tool can provide users with many examples of incident reports containing specific construction related concepts, so they can gain insight about the hazards associated with, say, equipment and activities that they are involved with.”
“This will transform the way we use incident and accident data. It will allow health and safety professionals and others to harness a vast range of experience almost effortlessly. This will help industry come up with evidence-based solutions to prevent harm from happening in the future.”
The tool holds the key to much richer, more effective searching that will have an impact for everyone concerned with safety in the construction industry. Sophia explains how human knowledge came together with powerful, intelligent processing to develop this ground-breaking new system:
“We worked with experts from the HSE, using sample documents which we annotated with rich semantic information. A small sample of documents was initially marked up manually using NaCTeM’s annotation environment by HSE experts and then used to train NaCTeM’s deep learning models– effectively teaching it to become a health and safety expert.”
In building the system, searching was enriched with annotations and content terms based on the HSE’s unrivalled knowledge of safety in the construction industry. Then labels were assigned related to broad categories such as hazards, materials, equipment, physical environment, environmental conditions, body part injured, construction activity, etc. These types were further refined – hazard sub-types include ‘public protection issue’, ‘struck’, ‘welfare issue’, etc. This fine-grained information enriches the document collection and is automatically extracted.
As well as helping the construction industry develop a much faster, data-driven approach to risk assessment, HSEarch has the potential to bring wider benefits for the HSE and for the ‘bigger picture’ of avoiding harm at work. “By analysing the unstructured text in other types of health and safety reports, text mining tools based on state of the art natural language processing techniques will enable us to easily access the wealth of knowledge of our inspectors,” says Tim. “Each inspection report is written by an inspector applying their knowledge and experience to a specific scenario. Text mining allows us to analyse reports collectively rather than individually.”
“By extending the capabilities of the tool we hope to go much further in exploring the reasons behind accidents and incidents, perhaps with an intelligent recommender system that links activities, equipment types etc. So, if you’re looking for information on harm that results from people falling from scaffolding, the system might also suggest that you look at harm resulting from objects falling too.”
The system behind the RIDDOR Text Analysis Tool can be tailored for use in any industry. The next steps will include engaging with construction companies to develop the system further, then taking it wider, to other industries. Discovering Safety would like to hear from people in construction and in other industries who want to explore the potential of text mining in improving safety. If you would like to get involved, please contact discoveringsafety@hse.gov.uk.
Click on the video below to watch Emrah Inan, Paul Thompson and Sophia Ananiadou (Manchester University) and Tim Yates (Health and Safety Executive) introduce the new semantic search system in our Technical Showcase.
If you think that using semantic search capabilities could help your industry, please get in touch with Sophia:
Sophia.ananiadou@manchester.ac.uk
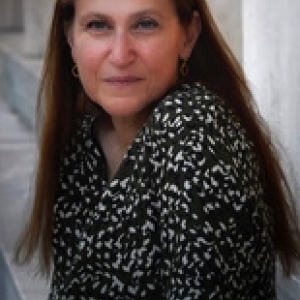
Related Content