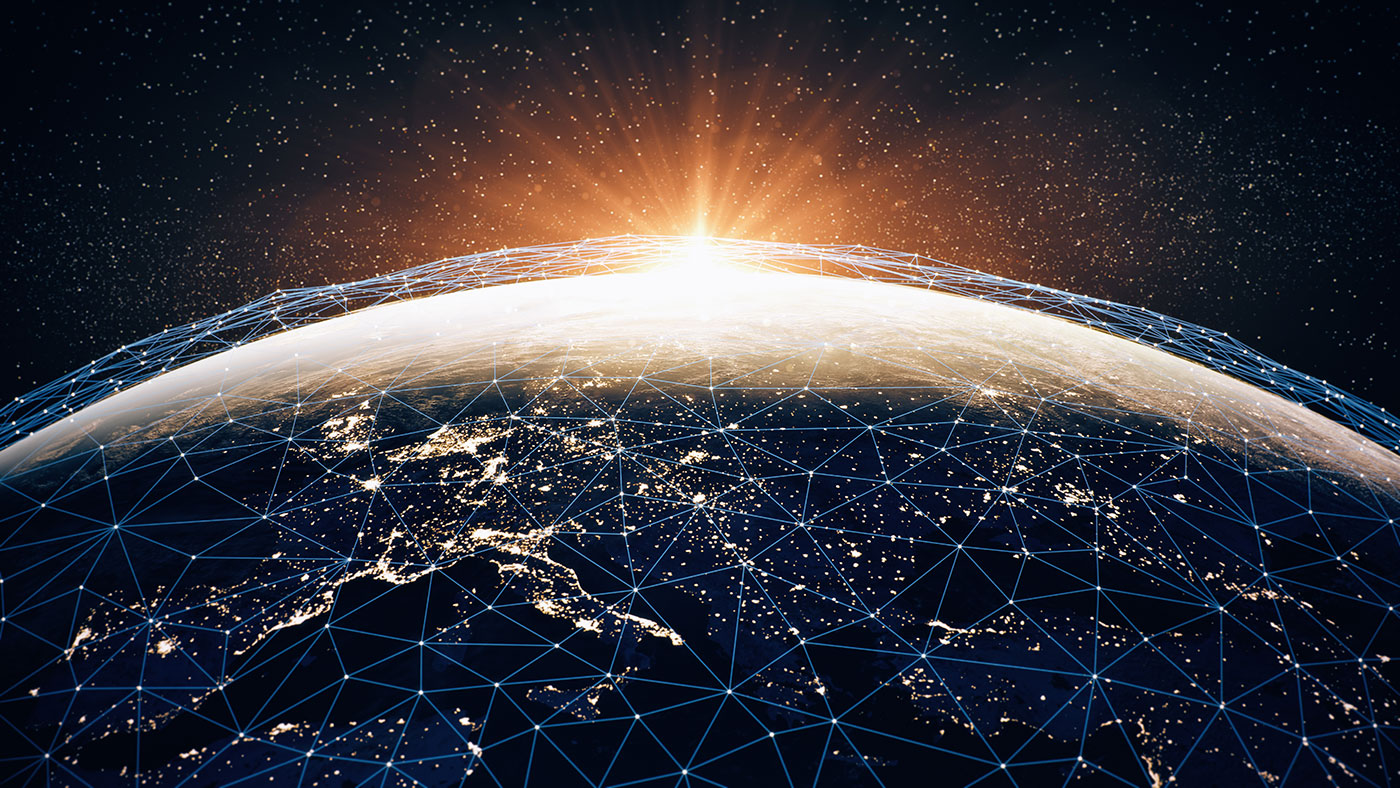
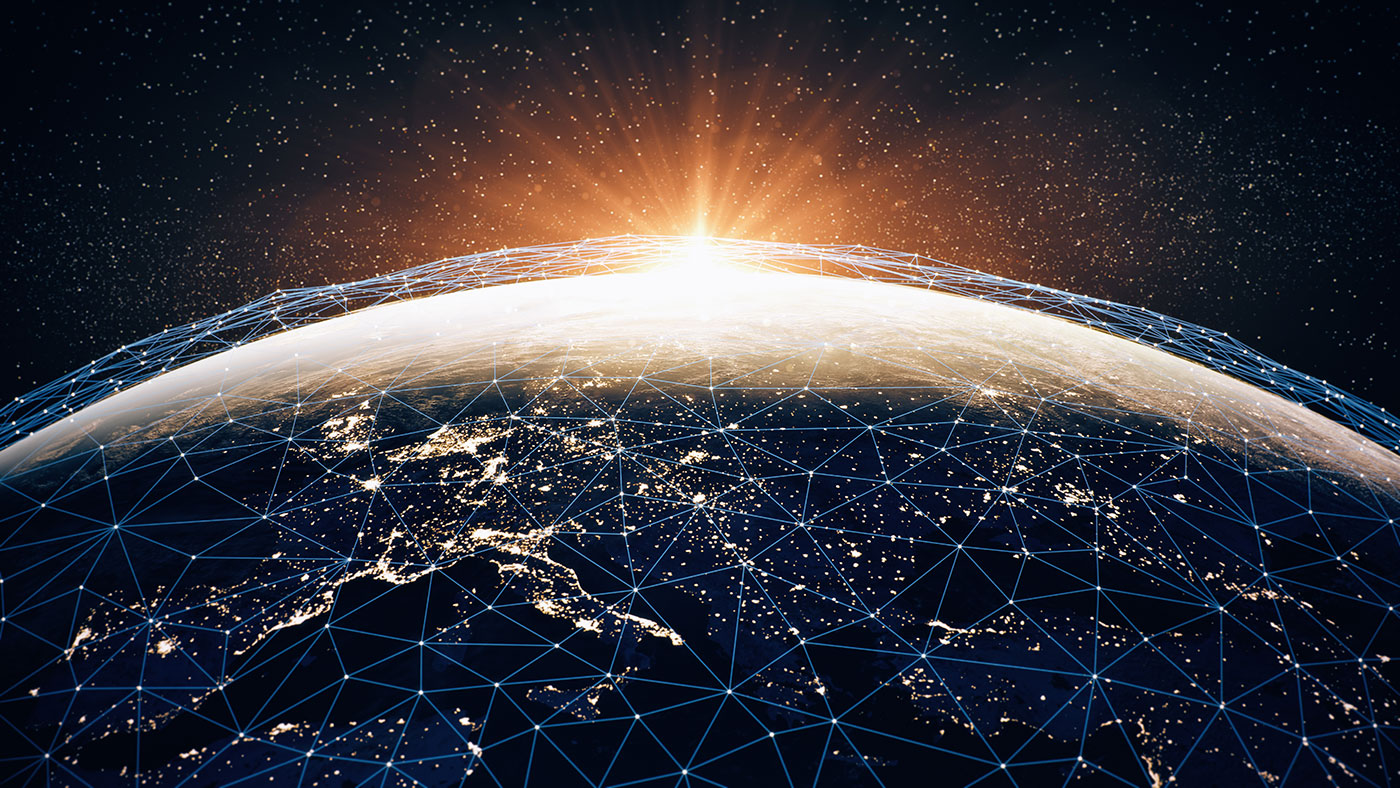
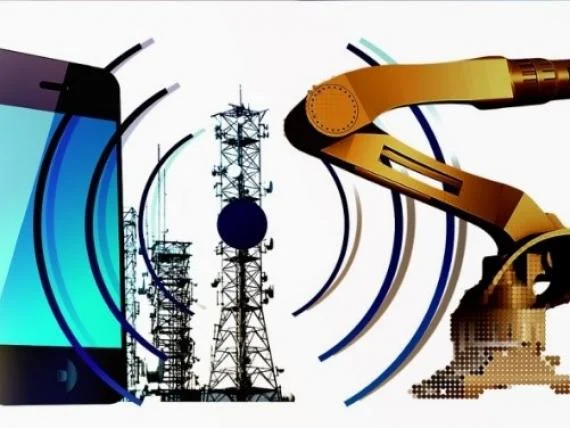
This series of six blogs considers key implications of the industry 4.0 revolution for health and safety, and practical challenges likely to be faced by organisations when looking to exploit industry 4.0 technologies to deliver improvements in operating performance, including health and safety performance.
This blog, the second in the series, considers how the emergence of industry 4.0 technologies and its use in parallel with artificial intelligence and machine learning is heralding a major shift in how health and safety is practiced in modern workplaces, enabling a much greater focus to be placed on prevention of future accidents, rather than learning from past ones.
In the previous article, I considered some of the key implications of the industry 4.0 technological revolution for health and safety and the aspirations for organisations to deliver continuous improvements in health and safety performance. The rise in use of industry 4.0 technologies and rise in use of AI based technologies within industrial operations are intimately linked. The data flows that characterise the functioning of industry 4.0 technologies provide the foundations for being able to use AI to support and even directly control the actioning of industrial tasks. The desired endpoints in using each technology are the same, namely the delivery of more efficient industrial operations.
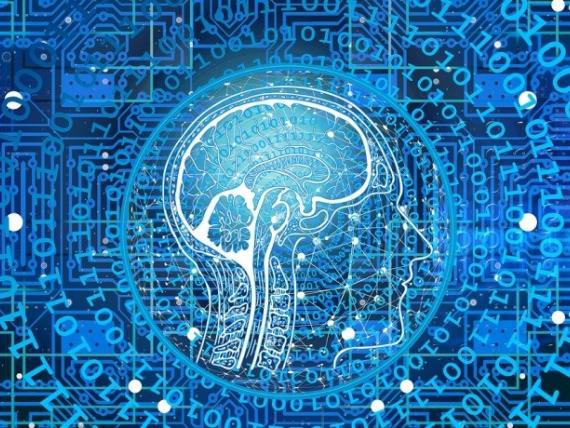
The focus of this article is on the AI dimension of industry 4.0; a perspective is offered on how the emergence of industry 4.0 and AI in parallel, is opening up opportunities for more proactive industrial decision-making, specifically in relation to how health and safety is practiced.
The research domain of artificial intelligence is a very fertile and diverse area of R&D currently, tapping expertise across numerous disciplines to address specific research challenges, including computer science, mathematics, statistics and data science, to name just a few. These disciplines operate in a rich terminological space, with terms often used loosely and interchangeably. For this reason it is felt useful as part of this article to start by offering a number of definitions of commonly used terms.
Artificial intelligence (AI) may be defined as:
“…the theory and development of computer systems able to perform tasks normally requiring human intelligence…”
Machine learning (ML), a branch of AI, may be defined as:
“…the science of getting computers to act without being explicitly programmed…”
At its most simplistic, a computer may act through the application of simple rules based logic written into a computer programme. The central challenge in machine learning is to train a computer algorithm to act autonomously without the need for explicit rules based logic. A common challenge area a ML algorithm is tasked to perform is to predict an outcome based on past occurrences of the outcome or co-occurrence of related factors, the central challenge being to predict the outcome as accurately as possible. This can naturally be undertaken relatively easily, simply by comparing the computer algorithm’s predictions to what actually happened in reality. Statistics can also be used to undertake such predictive tasks, as an alternative to using machine learning based techniques. Statistics may be defined as:
“…the science of collecting and analysing numeric data in large quantities, especially for the purpose of inferring proportions in a whole from those observed in a representative sample…”
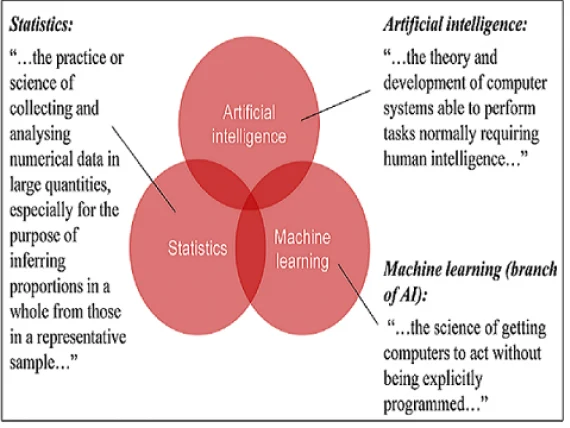
The general process by which a machine learning algorithm is trained to predict an outcome of interest and then is subsequently operationalised is illustrated in the Figure below. The process starts by defining the objective function for the AI system, that is, the specific outcome that the system is being trained to predict. A dataset is then compiled made up of variables characterising the outcome to be predicted, along with potential predictors. In this dataset, termed the training set, the relationship between the outcome and its predictors is known. The training set is split into a set used to train the algorithm to predict, and a second set used to test how well the final trained algorithm performs. The training process is typically a sequential, cyclical process of repeated training, validating, tuning and revalidating of the algorithm using different portions of the training set. In this way, the way the algorithm predicts is repeatedly refined and the way it predicts is repeatedly validated, which continues until a desired level of predictive performance is achieved. As the relationship between the outcome and predictors is known in the training set, the predictive accuracy of the algorithm can be measured at each stage of the validation process and the effects of algorithm tuning on predictive accuracy judged. The final performance of the algorithm is then measured on a final test set of data. If the predictive performance of the algorithm is deemed sufficiently accurate then the final step in the process is to deploy it operationally, feeding in new unlabelled data on a routine basis.
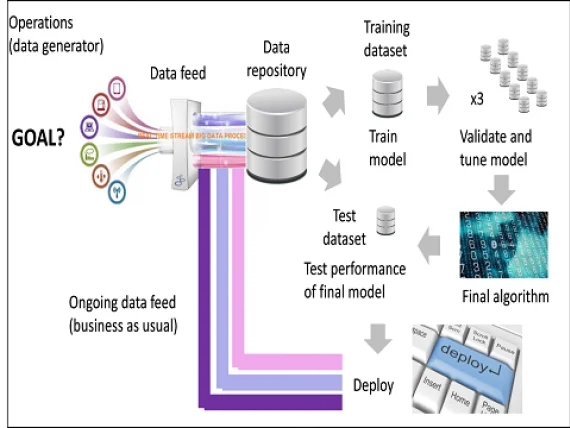
Examples of industrial use of ML are already numerous across many organisations today. For example, many automated process control systems used across process industries are increasingly using deep learning algorithms as part of the way they operate. In addition, enterprise asset management systems are increasingly making use of ML techniques to direct decisions relating to when assets are serviced and checked to maximise up-time and minimise down-time. Examples of more direct use in health and safety contexts are also increasingly commonplace. For example, major hazards industries are starting to use video analytic techniques as part of worksite access control systems, to ensure that workers are using appropriate personal protective equipment when starting work. Use of AI and ML technologies to support health and tasks of a more cognitive nature, for example, the sourcing of advice to ensure regulatory or standards compliance or recognised industry codes of practice are being followed, is also growing across a number of sectors.
A future article will consider future opportunities on the horizon, for example, the emerging interest in using AI based technologies in workplaces in conjunction with aerial drone technology to help in the structural inspection of industrial assets, CCTV technology to auto-detect unsafe working practices and precursors of serious accidents real time, wearables technology to predict future ill-health endpoints in workers, and expert systems technology to help impart bespoke health and safety risk advice.
The third blog in this series, to be published next week, will explore other ways in which emerging technologies are being used across workplaces to support the practice of a much more proactive type of health and safety.
The potential for use of industry 4.0 technologies in parallel with artificial intelligence to magnify the opportunities for better health and safety, but also to lead to new risks requiring control, will provide the focus of later blogs.
If you have any comments having read this blog, feel free to share them below.
Related Content