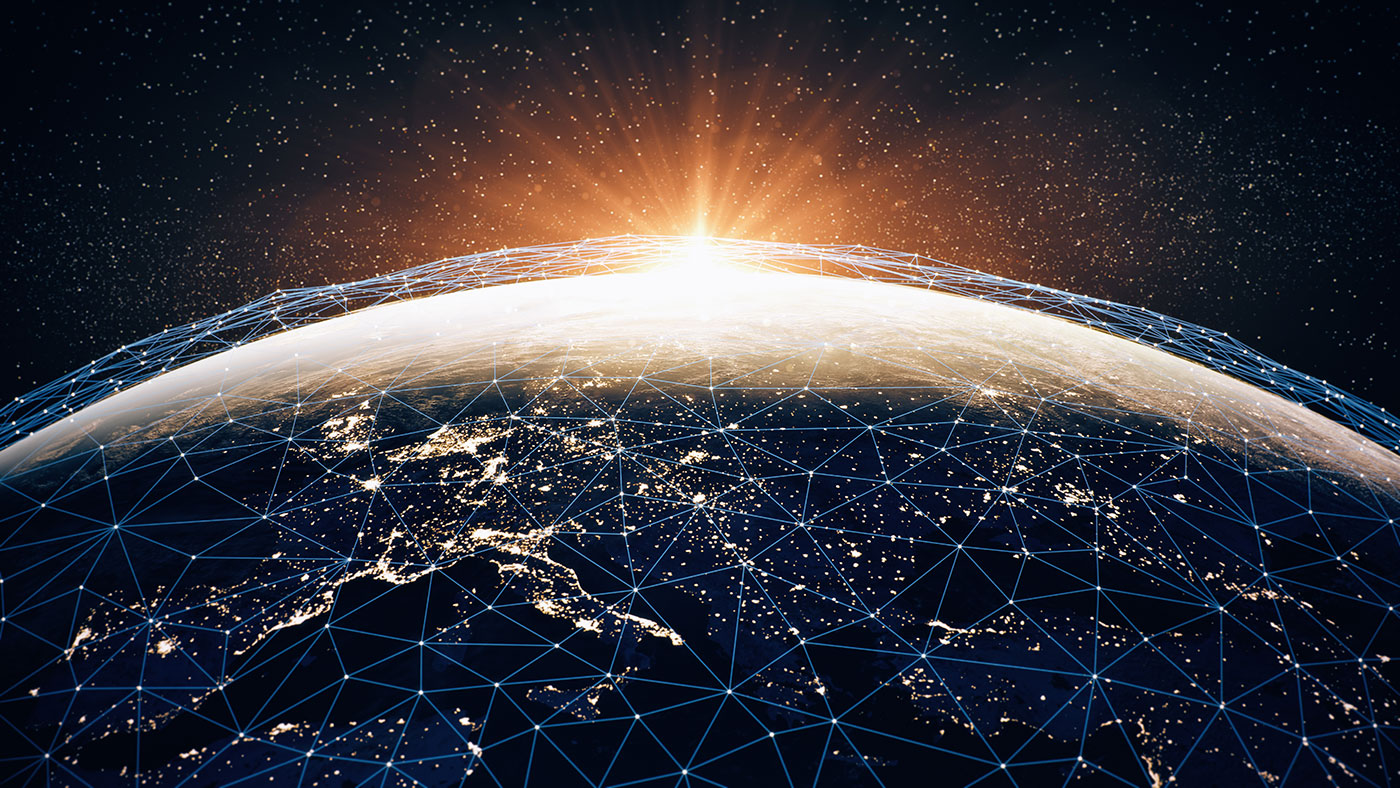
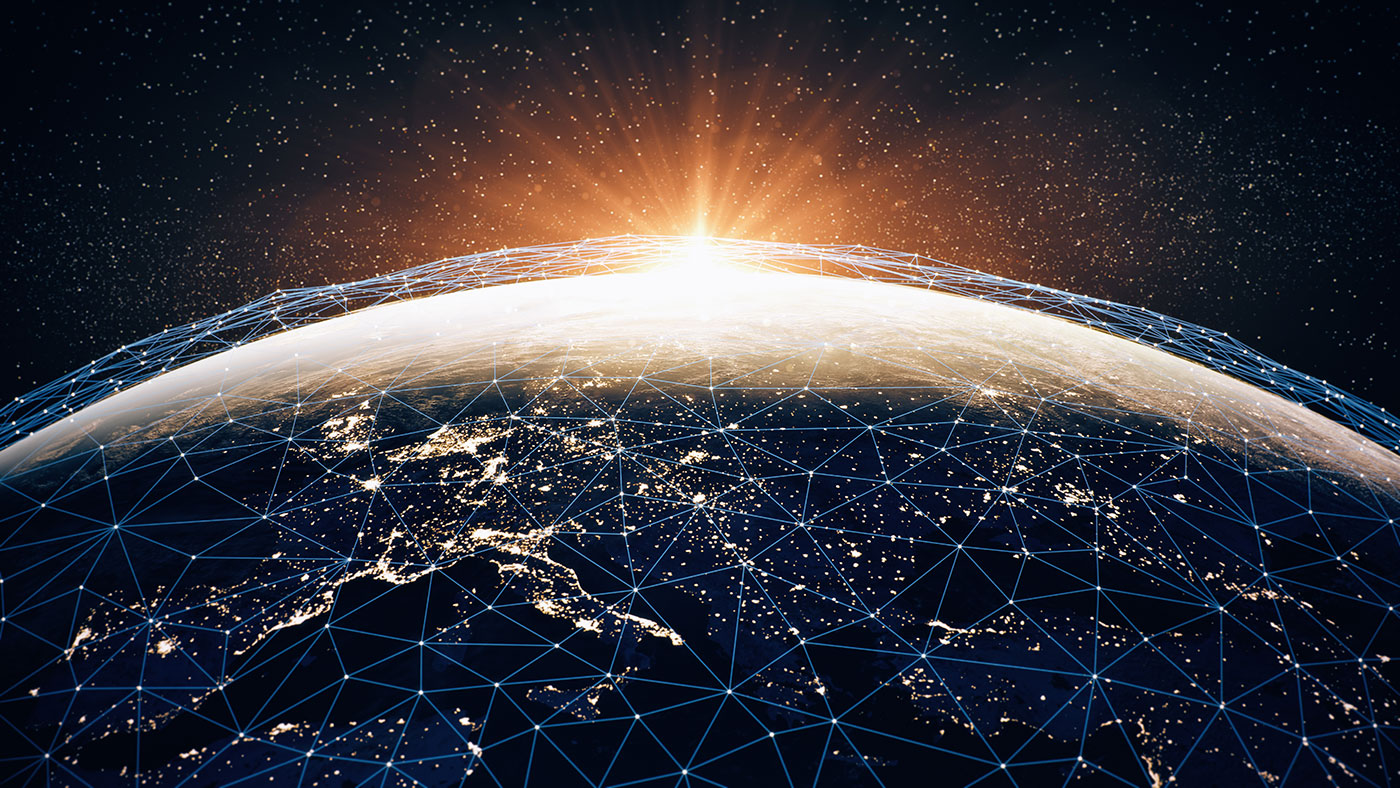
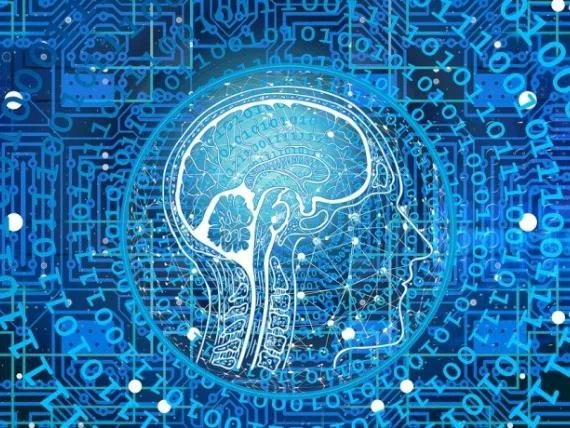
This is the first of the final 3 blogs discussing ‘Rise in industry use of AI technologies in workplaces and future challenges for health and safety’ in the series on Industry 4.0 and AI. In previous blogs, I considered future technological opportunities on the horizon for exploiting AI based technologies to deliver improvements in health and safety performance, both from a dutyholders and regulators perspective. In this article I consider potential knock on effects for health and safety in the event that AI systems get it wrong; I also offer a perspective on key considerations in attempting to mitigate such risks, and potential implications for future health and safety practice.
In considering different categories of AI, it is useful to view them on a continuum of increasing functional complexity, as in Figure 1. The continuum starts on the far left with simple rules based algorithms, moves on to inferential statistics for prediction, then onto machine learning based and then deep learning based prediction. The latter two techniques are examples of what is often termed as artificial narrow intelligence (ANI). ANI refers to AI based systems developed and trained for a specific task within a limited context. All AI based technologies currently being used operationally in workplaces are of this type.
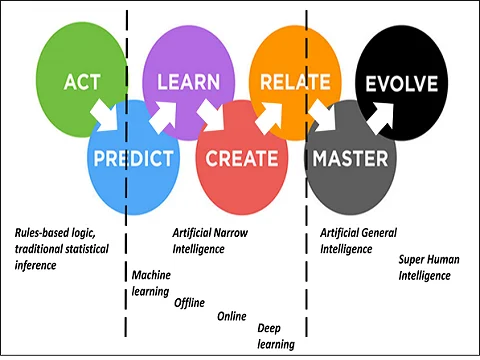
The emergence in use of deep learning algorithms within AI based technologies has resulted in step changes in the functional capabilities of systems. The terms machine learning (ML) and deep learning (DL) are often used interchangeably. However, the two techniques are subtly different in a number of ways. Both involve algorithms that 1) parse data, that is, analyse data in order to understand its content and structure, 2) learn from the parsing process, and then 3) apply what they’ve learned. However, DL refers to the use of a particular category of algorithm in the process known as an artificial neural network (ANN). A key difference between ML and DL is that the former requires a certain level of manual intervention from an analyst to inform the learning process, specifically the definition of the variables or features to be used in the learning process. In the latter however, the definition of the variables or features is self-directed by the algorithm. These differences are illustrated in Figure 2; the typical unit structure of an ANN, how it operates and its likeness to a human neurone is illustrated in Figure 3.
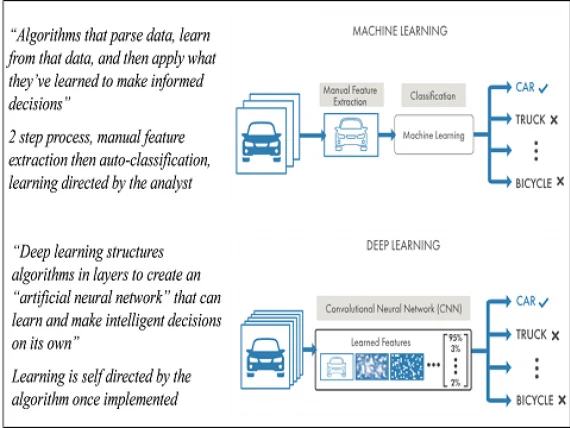
As technological advances continue, both in AI and ML and the computing power needed to fuel it, many futurists regard it as inevitable that artificial narrow intelligence systems will be replaced by wider, more general intelligence systems. This is obviously contingent on progress continuing unchecked. Artificial general intelligence (AGI) systems can be defined as AI systems with broad, adaptable cognitive abilities, similar to humans. On the ML continuum in Figure 1, ANI can be considered to be replaced by AGI at the point where algorithms transition from merely performing to actually mastering a particular task. Such algorithms can be characterised by possessing the capability of constructing abstract concepts, and transferring and applying them from one domain to another, as humans are able to do.
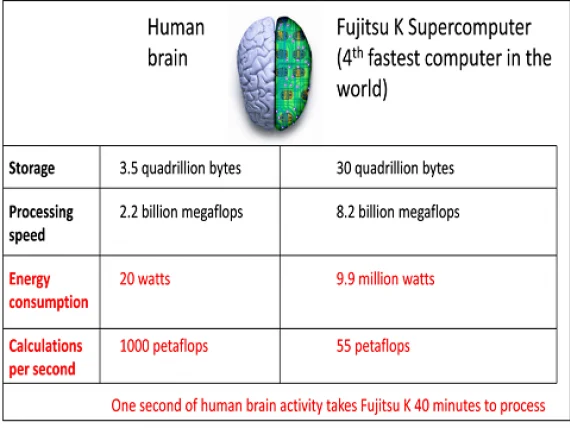
The emergence of AGI is no longer confined to the realms of science fiction, a recent survey of prominent AI experts found that consensus opinion was that AGI would be more likely than not realised by 2100. The capacity of the fastest supercomputers to store information is already around ten times greater than the human brain, whilst the speed with which information is processed is around four times greater. However, the number of calculations each second that the fastest supercomputer can currently manage is dwarfed by the human brain; the capacity of the human brain is twenty times greater in this regard; placing the latter in context, one second of human brain activity would take the fastest supercomputers around forty minutes to process (see Figure 4); computers therefore still have quite a way to go to match human brain power on this metric.
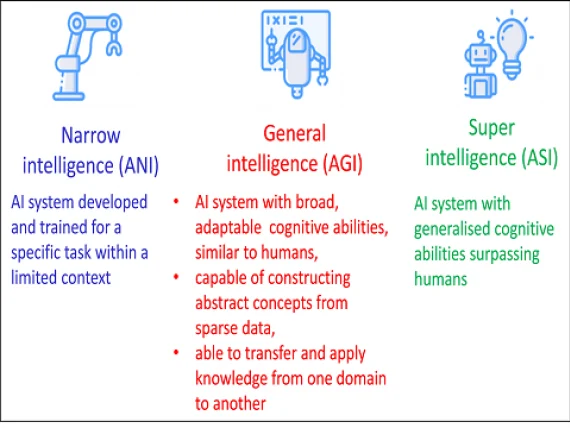
The final point on the far right of the ML continuum in Figure 1 is represented by artificial super intelligence (ASI), which may be described as AI systems with general cognitive abilities surpassing humans. Whereas humans are essentially fixed entities in time, super intelligence might be conceivably achieved through the emergence of entities that are able to change their own architecture and design instantaneously to adapt to changing needs. This however is currently the realms of science fiction.
Progression along the ML continuum paints an attractive picture of the future direction of travel of AI technological capabilities. However, this is obviously contingent on AI systems consistently making the right decisions on our behalf. Realising this requires careful design of the technologies in the first instance, along with careful deployment and ongoing use. However, there have been a number of high profile examples in recent years of AI systems getting it wrong and more general concerns voiced over how the technology is looking to be used in different contexts. One such example is Uber’s Volvo XC90 self-driving car, which, whilst on a test driven in the US, collided with a pedestrian, fatally injuring them, after failing to stop whilst the pedestrian was crossing a road; a second example of similar such systems getting it wrong is the two Boeing 737 Max 8 aircraft crashes, one in Indonesia and a second six months following in Ethiopia, where a fault involving an algorithmically controlled anti-stall system was implicated in each accident. Other examples, albeit non-safety related, include Microsoft’s Tay chatbot, a social experiment where a computer program trained with the personality of a typical 19 year old was posted on Twitter and other social media platforms to chat with human users; however, the bot ended up having to be taken down from the sites after only a day after developing a shockingly racist personality; PredPol, a computer system used by a number of police depts. across the US that uses archived data on past criminal activity and related factors to predict the likelihood of future crimes taking place in specific localities to inform the targeting of policing; however PredPol has been criticised by some for being racially biased; and Compas, a computer system used by a number of criminal courts across the US that uses archived data on the circumstances of past prisoner reoffending to predict the likelihood of future reoffending to support parole decisions; however, again, its decisions have been criticised by some for being racially biased.
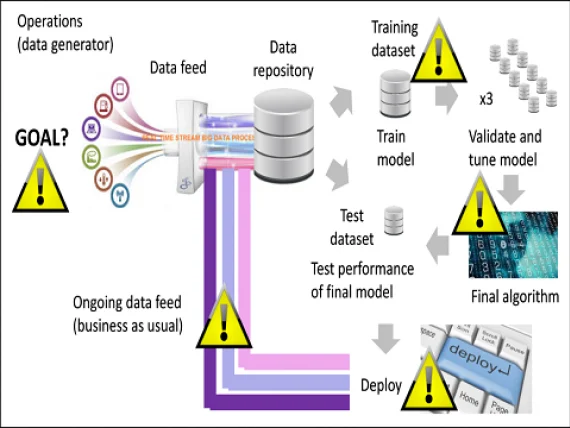
The above examples provide useful lessons learned, elucidating on the different ways in which AI systems might conceivably go wrong. In exploring these routes to failure in more detail, it’s useful to first consider the general process by which a machine learning algorithm is trained to predict an outcome of interest and then is subsequently operationalised; this is done in Figure 5. There are a number of places in the process depicted in Figure 5 where a systematic error might result in an inaccurate end prediction and a subsequent undesired, potentially deleterious outcome. One potential source of error is where the objective function, so defined, leads to an unforeseen adverse outcome when the AI system is subsequently deployed; a second is where the training set compiled does not provide an accurate representation of the true relationship between the outcome and predictor variables; a third is where there is an error with the model itself; a fourth is where there is an error with the business as usual data feed; and a fifth is an error with how the model outputs are translated into subsequent operational decisions.
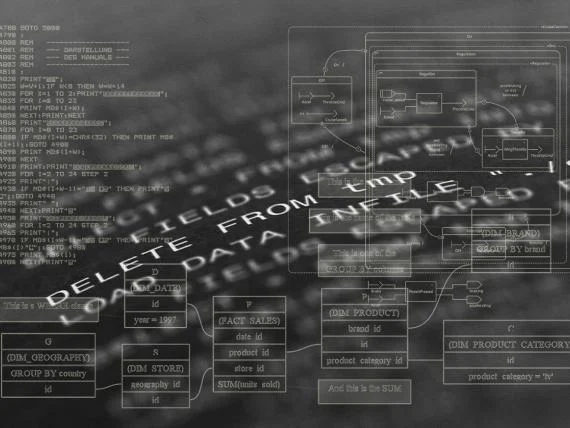
Any strategy aiming to address the risks of AI systems getting it wrong obviously needs to give due consideration to all such potential routes of failure to ensure that the AI system’s goals remain aligned with actual desired goals, whether they be health and safety related or otherwise. Algorithmic transparency is obviously an essential prerequisite for being able to do this. Periodic checking of the objective function and assessment of the risks of any unintended knock-on effects is key to minimising risks associated with the first failure route in Figure 5; for the second failure route, periodic checking and renewal of the training set at adequately timed intervals to ensure that it adequately mirrors the objective function; for the third, periodic retraining of the algorithm at adequately timed intervals and testing for overfitting and associated errors; for the fourth, periodic checking of data inputs to the model once deployed; and for the fifth, periodic checking of model data outputs and how they are translated into subsequent operational decisions, particularly if this step is automated also.
The direct technical challenge of striving to ensure that an AI system’s goals are aligned with actual desired goals is not the only category of challenge faced when thinking about the implications of the rise in industrial use of AI for health and safety. Others abound, including the legalities when AI systems go wrong and workers are injured or worse, the challenges faced by health and safety regulators when tasked with investigating such failures, or judging whether risks are sufficiently well mitigated, the ethics of using AI systems in different contexts and dealing with industrial cyber risks in the industry 4.0 era. These areas will provide the focus in a future article.
Watch our for part 2 of ‘Rise in industry use of AI technologies in workplaces and future challenges for health and safety’, which will be released later this week.
Related Content